Microfossil classification plays a crucial role in subsurface exploration for oil & gas and Carbon Capture and Storage (CCS). These microscopic fossils found in sedimentary rocks provide valuable information about the age and depositional environment of geological formations. Traditionally, this analysis has been a labor-intensive process performed by human experts. However, recent advancements by the machine learning group at the University of Tromsø (UiT), The Arctic University of Norway, promise to revolutionize this field.
Challenges in Automating Microfossil Analysis
Automating microfossil classification presents two primary challenges:
- Massive Data Volume: The dataset of microfossils is immense, projected to grow to 3 billion specimens.
- Lack of Labeled Data: There is insufficient labeled data to train a deep learning classifier using standard methods.
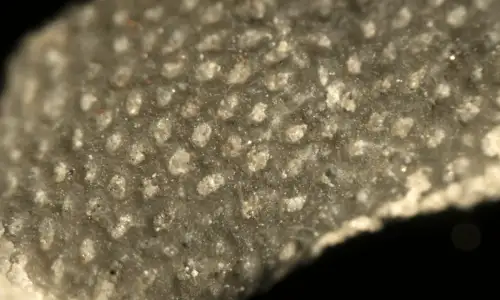
Innovative Solutions with Self-Supervised Learning
The UiT machine learning team has developed an efficient pipeline for processing and grouping fossils by genus or species using self-supervised learning. Their approach involves several key steps:
- Efficient Crop Extraction: They adapted previously trained object detection algorithms to efficiently extract crops from whole slide images.
- Self-Supervised Learning Methods: A range of self-supervised learning methods were compared to classify and identify microfossils with minimal labeled data.
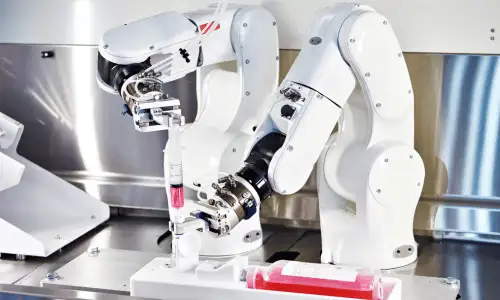
Breakthrough Results
“This work shows that there is great potential in utilizing AI in this field,” says Iver Martinsen, first and co-corresponding author of the study. The AI developed by the UiT team demonstrates the ability to automatically detect and recognize fossils, significantly aiding geologists in making better use of wellbore samples.
Microfossils are found abundantly, but the expertise and time required for analysis have limited the extent of their study. By leveraging state-of-the-art AI methodologies, the UiT researchers trained an AI model without annotations, utilizing a vast pool of raw data provided by the Norwegian Offshore Directorate.
Training and Testing the Model
The AI was used to detect fossils from a selected well on the Norwegian continental shelf. The model was trained on 100,000 detected fossils and its performance was evaluated by classifying several hundred labeled fossils from the same well. The results were impressive, with the AI model exceeding previous benchmarks in microfossil analysis.
Both convolutional neural networks and vision transformers fine-tuned by self-supervision delivered excellent results. This approach proved to be fast and computationally light, offering a valuable tool for geologists working with microfossils.
Implications for Industry and Academia
The advancements made by the UiT machine learning group have significant implications for both industry and academia. By automating the labor-intensive process of microfossil classification, this AI technology can help geologists better utilize the enormous amount of information provided by wellbore samples. This can lead to more accurate and efficient subsurface exploration and monitoring, benefiting sectors such as oil & gas and CCS.